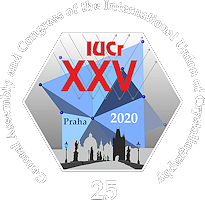
XXV General Assembly and Congress of the
International Union of Crystallography - IUCr 2021
August 14 - 22, 2021 | Prague, Czech Republic
Conference Agenda
Overview and details of the sessions of this conference. Please select a date or location to show only sessions at that day or location. Please select a single session for detailed view (with abstracts and downloads if available).
|
Session Overview |
Session | |
Poster - 07 Powder: Powder diffraction - general, structure refinement
| |
Presentations | |
Poster session abstracts Use of intermolecular distances from ssNMR in crystal structure determination from powder diffraction data 1Institute of Physics of the Czech Academy of Sciences, Na Slovance 2, Prague 8, 182 21, Czech Republic; 2Institute of Macromolecular Chemistry, Czech Academy of Sciences, Heyrovsky Square 2, Prague, 16206, Czech Republic; 3Department of Solid State Chemistry, University of Chemical Technology Prague, Prague 6, 166 28, Czech Republic The steady-state in the field of software allowing the application of different approaches for crystal structure determination [1,2] could give the impression that the determination of the crystal structure from the powder diffraction data is a common and straightforward task that does not deserve additional attention. However, looking at crystal structures determined from powder diffraction data, one can estimate the limit of the method. In the words of degrees of freedom, the current limit is around 40. The two most complex crystal structures found in the CSD database [3,4], and also many others, were solved using the direct-space methods [5]. Simply said, the direct-space methods find the structural model by changing the position and shape of the molecular fragments in the asymmetric part of the unit cell. They allow defining additional conditions for the studied crystal structure, which is handled as additional observation. For example, the model can be restricted by several specifying torsion angles or by rigid groups as it is already implemented in existing software [6,7]. All these additional observations aim to make it possible to find a solution or at least significantly reduce the calculation time. Another observation that may increase the probability of finding the correct solution is the information about intermolecular distances in the crystal structure. This information can be obtained by performing a specific ssNMR measurement which usually offers a list of short-range interactions between atoms. We decided to implement such a possibility to the already existing software FOX [5], and we tested it on several compounds. First of all, we tested it on the already solved crystal structures. We defined intermolecular distances between several selected atoms with various precisions, and we used them as additional restrictions that influenced the final cost function. We then tested it on a compound with an unknown crystal structure, for which we obtained estimated intermolecular distances from the ssNMR. We used these additional observations for the structure solution process from X-ray powder diffraction data. 1. David, W.I.F.; Shankland, K. Structure Determination from Powder Diffraction Data. Acta Crystallogr. A 2008, 64, 52–64, doi:10.1107/S0108767307064252. 2. Meden, A.; Radosavljevic Evans, I. Structure Determination from Powder Diffraction Data: Past, Present and Future Challenges: Structure Determination from Powder Diffraction Data: Past, Present and Future. Cryst. Res. Technol. 2015, 50, 747–758, doi:10.1002/crat.201500048. 3. Husak, M.; Jegorov, A.; Czernek, J.; Rohlicek, J.; Zizkova, S.; Vraspir, P.; Kolesa, P.; Fitch, A.; Brus, J. Successful Strategy for High Degree of Freedom Crystal Structure Determination from Powder X-Ray Diffraction Data: A Case Study for Selexipag Form I with 38 DOF. Cryst. GROWTH Des. 2019, 19, 4625–4631, doi:10.1021/acs.cgd.9b00517. 4. Fernandes, P.; Shankland, K.; Florence, A.J.; Shankland, N.; Johnston, A. Solving Molecular Crystal Structures from X-Ray Powder Diffraction Data: The Challenges Posed by γ-Carbamazepine and Chlorothiazide N,N,-Dimethylformamide (1/2) Solvate. J. Pharm. Sci. 2007, 96, 1192–1202, doi:10.1002/jps.20942. 5. Černý, R.; Favre-Nicolin, V. Direct Space Methods of Structure Determination from Powder Diffraction: Principles, Guidelines and Perspectives. Z. Für Krist. - Cryst. Mater. 2007, 222, doi:10.1524/zkri.2007.222.3-4.105. 6. Černý, R.; Favre-Nicolin, V.; Rohlíček, J.; Hušák, M. FOX, Current State and Possibilities. Crystals 2017, 7, 322, doi:10.3390/cryst7100322. 7. David, W.I.F.; Shankland, K.; van de Streek, J.; Pidcock, E.; Motherwell, W.D.S.; Cole, J.C. DASH : A Program for Crystal Structure Determination from Powder Diffraction Data. J. Appl. Crystallogr. 2006, 39, 910–915, doi:10.1107/S0021889806042117. External Resource: https://www.xray.cz/iucrp/P_178
Structure determination of soft crystal polymorphs by using prediction technique with powder X-ray diffraction data 1CONFLEX Corporation, Tokyo, Japan; 2Information and Media Center, Toyohashi University of Technology, Toyohashi, Japan Soft crystals transform into another polymorphic forms by macroscopic gentle stimuli at room temperature and show remarkable changes in luminescence and optical properties [1]. In order to control and analysis the phenomena of soft crystals, it is important to determine the polymorphs and clarify mechanism of the polymorphic transitions. We have developed computational techniques for crystal structure prediction (CSP) and provided successful results in a past blind test of CSP [2]. Recently, we reported a new method related to the CSP technique with measured powder X-ray diffraction (PXRD) data [3]. The method can find the observed crystal structure among a number of computationally suggested structures by using the measured PXRD data and crystal energy, while it is often difficult to find that by only an energy evaluation even with advanced calculations. Therefore, the method can provide candidate structures to experimental crystal structure analyses of unknown crystal structures from the PXRD data. In this presentation, this new method is applied to flexible organic molecules including the soft crystal materials where the structure analysis from the PXRD data is often difficult, and it is demonstrated that the appropriate crystal structures can be determined by using the CSP technique with PXRD data. We also show that this method is useful for polymorphism analysis. [1] Kato, M., Ito, H., Hasegawa, M. & Ishii, K. (2019). Chem. Eur. J. 25, 5105. [2] Reilly, A. M. et al. (2016). Acta Cryst. B72, 439. [3] Ishii, H., Obata, S., Niitsu, N., Watanabe, S., Goto, H., Hirose, K., Kobayashi, N., Okamoto, T. & Takeya, J. (2020). Sci. Rep. 10, 2524. External Resource: https://www.xray.cz/iucrp/P_177
Easy Automation & More Accurate Analysis with HighScore(Plus) V4. Malvern Panalytical B.V., Almelo, Netherlands, The Creating an automated XRPD analysis is often hampered by either a complex GUI (setting it up is difficult) or because the analysis itself is too complicated and requires decisions, loops or other non-linear elements. In our HighScore(Plus) V4.9 [1] release we have solved both obstacles at once by providing a graphical Flowchart alike design & execution Interface, that can contain decision steps as well as any number of loops. The automation batch is simply put together by dragging and connecting action and decision step boxes. In addition, supervised and unsupervised learning features are greatly improved, by adding the very popular t-SNE method [2] to cluster (neighborhood) analysis, and by enhancing the automatic optimization of pre-processings [3] and automated variable selection [4] to the PLSR [5,6] implementation. The cross-validation is sped up by a factor of 100 by using multi-threading and algorithm optimizations. All in all, these additions allow creating better, more accurate predictive models in a much shorter time. [1] T. Degen, M. Sadki, E. Bron, U. König & G. Nénert, The HighScore Suite, Powder Diffr. Vol. 29, (2014), Supplement S2, 13-18. [2] L.J.P. van der Maaten and G.E. Hinton, Visualizing High-Dimensional Data Using t-SNE. Journal of Machine Learning Research 2008, 9, 2579-2605. [3] Jan Gerretzen, Ewa Szymańska, Jeroen J. Jansen, Jacob Bart, Henk-Jan van Manen, Edwin R. van den Heuvel, Lutgarde M. C. Buydens, Simple and Effective Way for Data Preprocessing Selection Based on Design of Experiments, Anal. Chem. (2015), 87, 12096-12103. [4] Loann David Denis Desboulets, A Review on Variable Selection in Regression Analysis, Econometrics 2018, 6(4), 45 [5] Wold, Herman, (1966). Estimation of principal components and related models by iterative least squares, in P.R. Krishnaiaah, Multivariate Analysis. New York: Academic Press. (1966), pp. 391-420. [6] S. de Jong, SIMPLS: An alternative approach to partial least squares regression, Chemometrics Intell. Lab. Syst., (1993), 18(3), 251-263. External Resource: https://www.xray.cz/iucrp/P_180
Structural characterization of MgxCo3-xP2O8 solid solutions 1University of Oviedo, Oviedo, Spain; 2Jaume I University, Castellon, Spain Electrical, optical and magnetic properties of phosphates related in the literature made interesting the study of these materials. Specifically, many structures of phosphates are stables at high temperature [1]. Co(II) phosphates avoid some deficiencies detected when cobalt oxides or other cobalt salts are used as raw materials in the synthesis of ceramic pigments [2]. Cobalt violet phosphate, Co3P2O8, is included in the DCMA Classification of the Mixed Metal Oxide Inorganic Coloured Pigments (DCMA-8-11-1) [3]. Melting point in Co3P2O8 compound at 1160 ºC could be increased from the formation of solid solutions with Mg3P2O8 with melting point at 1240 ºC. The stable polymorph of Co3P2O8 compound presents Mg3P2O8 structure with monoclinic symmetry, so the formation of these solid solutions seems possible in a partial or total compositional range. Unit cell parameters are a = 5.064 Å, b = 8.371 Å, c = 8.794 Å, β = 121.01 in Co3P2O8 compound (ICSD-38259) and a = 5.077 Å, b = 8.230 Å, c = 8.833 Å, β = 120.94 in Mg3P2O8 compound (ICSD-31005). The variation of the unit cell parameters with composition will confirm the formation of these solid solutions. Structural characterization of the MgxCo3-xP2O8 compositions with temperature, position of the Co(II) absorption bands in Visible spectrum and measurement of the CIEL*a*b* colour parameters [4] give us information about the composition and temperature in which the desired colour is developed. Figure 1. Rietveld refinement from Co3P2O8 composition fired at 1000 °C (L* = 32.12, a* = +25.99, b* = -25.56) [1] Tena, M. A., Mendoza, R., García, J. R. & García-Granda, S. (2017). Results in Physics, 7, 1095-1105. [2] Tena, M. A., Mendoza, R., Trobajo, C., García, J. R. & García-Granda, S. (2018). J. Am. Ceram. Soc., 00, 1-10. [3] Dry Color Manufacturer’s Ass. (1982). DCMA Classification and Chemical description of the Mixed Metal Oxide Inorganic Coloured Pigments. 2nd ed. Metal Oxides and Ceramics Colours Subcommittee, Washington DC. [4] Commission Internationale del’Eclairage (1971) Recommendations on Uniform Color Spaces, Color Difference Equations, Phychometrics Color Terms. 1978. Supplement nº 2of CIE Publication Nº 15 (E1-1.31). Bureau Central de la CIE, Paris We gratefully acknowledge the financial support provided by Spanish Ministerio de Ciencia, Innovación y Universidades, project MAT2016-78155-C2-1-R. External Resource: https://www.xray.cz/iucrp/P_179
|