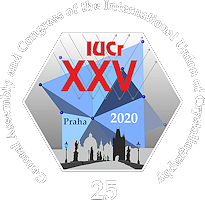
XXV General Assembly and Congress of the
International Union of Crystallography - IUCr 2021
August 14 - 22, 2021 | Prague, Czech Republic
Conference Agenda
Overview and details of the sessions of this conference. Please select a date or location to show only sessions at that day or location. Please select a single session for detailed view (with abstracts and downloads if available).
|
Session Overview |
Session | ||
MS-5a: Crystal structure prediction
Invited: Ivan Kruglov (Russia), Graeme Day (UK) | ||
Session Abstract | ||
For all abstracts of the session as prepared for Acta Crystallographica see PDF in Introduction, or individual abstracts below. | ||
Introduction | ||
Presentations | ||
10:20am - 10:25am
Introduction to session 10:25am - 10:55am
T-USPEX - novel method for crystal structure prediction at finite temperatures 1Dukhov Research Institute of Automatics (VNIIA), Moscow, Russia; 2Moscow Institute of Physics and Technology, Dolgoprudny, Russia; 3Skolkovo Institute of Science and Technology, Moscow, Russia; 4Northwestern Polytechnical University, Xi'an, China Due to the ever-increasing demands on the materials with enhanced properties, the task of searching for them using atomistic modeling methods is becoming increasingly important. The problem of designing novel materials comes down to finding a global minimum in a very noisy landscape in a multi-dimensional space (potential energy surface). This problem can be solved using several methods, yet the USPEX [1,2,3] evolutionary algorithm proved its effectiveness. The USPEX limitations are calculation at zero temperatures and small number of atoms in the unit cell, since the calculation of the energy of the structures (and their selection) takes place within the framework of the density functional theory (DFT). Here we present a new method (T-USPEX) which is capable of finding stable structures at finite temperatures and pressures. T-USPEX is based on the previously developed evolutionary algorithm USPEX. The main differences come from crystal structure relaxation at finite temperature and from the way of fitness function calculation (in this case – Gibbs free energy). Relaxation part is done using molecular dynamics in the NPT ensemble with pressure corrections taken into account. Gibbs free energy is calculated using thermodynamic integration with the corrections from thermodynamic perturbation theory. For these methods a big supercell is needed, so MTP [4] machine learning interatomic potentials is used. In this talk results for high-temperature phases of Al, Fe, Ti, U and MgSiO3 will be presented. Acknowledgments: This work was supported by RFBR foundation № 19-73-00237. [1] C. W. Glass, A. R. Oganov, and N. Hansen, Comput. Phys. Commun. 175, 713 (2006). [2] A. R. Oganov, A. O. Lyakhov, and M. Valle, Acc. Chem. Res. 44, 227 (2011) [3] A. O. Lyakhov, A. R. Oganov, H. T. Stokes, and Q. Zhu, Comput. Phys. Commun. 184, 1172 (2013). [4] A. V. Shapeev, Multiscale Model. Simul. 14, 1153 (2016) External Resource: https://www.xray.cz/iucrv/vidp.asp?id=413
10:55am - 11:25am
Functional materials exploration through evolutionary searching and large-scale crystal structure prediction University of Southampton, Southampton, United Kingdom The design of molecular crystals with targeted properties is the goal of crystal engineering. However, our predictive understanding of how a crystal’s properties relate to its structure, and how crystal structure in turn relates to molecular structure, are not yet sufficiently reliable to confidently design functional materials. Computational methods for crystal structure prediction (CSP) have been developed to help anticipate the crystal structure that a molecule will form. These methods are based on a global search of the lattice energy surface and a ranking of local energy minima according to their calculated relative stabilities. Thus, each molecule is associated with a list of potential crystal structures, each of which then leads to a set of predicted properties. The resulting ensemble of structures, their relative energies and associated properties can be interpreted to judge a molecule's promise for a target function. These methods have been demonstrated to be valuable in guiding experimental materials discovery programmes. A remaining challenge is the best choice of molecules that should be assessed, given the enormous chemical space of possible molecules. To address this, we have combined evolutionary searching of chemical space with large scale crystal structure and property prediction as a route to the discovery of novel molecules with high likelihood of yielding good properties [1]. The approach will be discussed with example studies in the area of organic semiconductor discovery. [1] Cheng, C. Y., Campbell, J. E. and Day, G. M. (2020) Chem. Sci.,11, 4922-4933 External Resource: https://www.xray.cz/iucrv/vidp.asp?id=414
11:25am - 11:45am
Design of metal-organic frameworks: from theoretical structure and reactivity predictions to mechanochemical synthesis and screening 1Faculty of Chemistry, University of Warsaw, Warsaw 02-093, Poland; 2Engineering Department, University of Cambridge, Cambridge CB2 1PZ, U.K; 3Department of Chemistry, McGill University, Montreal H3A 0B8, Canada; 4School of Metallurgy and Materials, University of Birmingham, Edgbaston, Birmingham B15 2TT, U.K. Metal-organic frameworks (MOFs) are microporous materials with many exciting applications, such as gas storage and separation, catalysis, platforms for artificial photosynthesis and energetic materials. The wide range of applications is strictly related to the modular node-and-linker composition, where different combinations of building blocks yield materials with various properties. The presence of a vast number of combinations for different node and linker, however, poses a real challenge for the experimental MOF design. An ab initio crystal structure prediction (CSP) method for MOFs has been reported by our group recently, and the method is based on the ab initio random structure searching (AIRSS) [1] and Wyckoff Alignment of Molecules (WAM) [2] algorithms. In this publication, a wide range of existing MOF structures have been investigated. Herein, we will demonstrate the first examples for the prediction of new MOF materials from metal azolate framework (MAF) and hexafluorosilicate families using our CSP method, combined with experimental mechanochemical synthesis and crystal structure determination. The solvent-free mechanochemical synthesis guided by theoretical structure prediction provides for an efficient and green approach to MOF design. The concept of MOF design goes beyond just the prediction of crystal structures. The connections between the crystal structures and chemical reactivity of freshly designed MOFs will also be studied by utilizing periodic density functional theory (DFT). Furthermore, our theory-based MOF structure and property predictions will be validated experimentally via mechanochemical screening and thermal studies, and ultimately aiming to improve our understanding of MOFs.
[1] Pickard, C. J.; Needs, R. J. (2011). J. Phys. Condens. Matter 23, 53201. [2] Darby, J. P.; Arhangelskis, M.; Katsenis, A. D.; Marrett, J. M.; Friščić, T.; Morris, (2020). Chem. Mater. 32, 5835–5844. External Resource: https://www.xray.cz/iucrv/vidp.asp?id=415
11:45am - 12:05pm
CCDCGAN: Deep learning prediction of crystal structures Institute of Materials Science, Technical University of Darmstadt, 64287 Darmstadt, Germany Autonomous materials discovery with desired properties is one of the ultimate goals for materials science [1]. In this work, we have developed constrained crystal deep convolutional generative adversarial networks (CCDCGAN, Figure 1(a)) based on a proper construction of the latent space [2], which can predict stable crystal structures. In particular, physical properties can be optimized in the latent space, where the formation energy is considered in the current model so that stable structures are predicted directly. We have successfully applied the approach on a randomly chosen binary Bi-Se system and observed that most known phases can be validated with quite a few distinct structures predicted [3]. Furthermore, trained using more than 50,000 compounds in the Materials Project database, we recently extended the algorithm to multicomponent systems coving most elements in the periodic table. As shown in Figure 1(b), two novel structures can be obtained for the Cd-Li system. Detailed analysis reveals that the approach can be used to predict novel crystal structures for various materials systems, and the generation efficiency can be further improved by considering a larger training set. It is expected that the other physical properties (such as band gaps) can be optimized in the latent space as well, giving us the chance to perform multi-objective optimization in the future. External Resource: https://www.xray.cz/iucrv/vidp.asp?id=416
12:05pm - 12:25pm
Computational design and prediction of solid-state properties of metal-organic frameworks 1University of Warsaw, Warsaw, Poland; 2Department of Engineering, University of Cambridge; 3Peter A. Rock Thermochemistry Laboratory and NEAT ORU, University of California Davis; 4Department of chemistry, McGill University; 55School of Metallurgy and Materials, University of Birmingham; 6School of Molecular Sciences and Center for Materials of the Universe, Arizona State University Design of new types of metal-organic frameworks (MOFs), the microporous materials with a wide range of functional properties, is an active area of materials research. The vast variety of available linker and node combinations leads to an incredible variety of potential MOF structures, providing an opportunity for tailoring the functional properties and thermodynamic stability of the new materials. At the same time, navigating the vast structural space of putative MOFs is proving to be a challenge for experimental screening, that can benefit from the guidance provided by computational chemistry methods. In this presentation we will describe the application of periodic density-functional theory (DFT) calculations together with state-of-the-art ab initio crystal structure prediction (CSP) calculations in elucidating the structural aspects of MOF thermodynamic stability and performing computational property-driven design of new MOFs. The presentation will commence with a theoretical study of the systematic effects of linker substituents on the thermodynamic stability of a series of isostructural zeolitic imidazolate frameworks (ZIFs) with sodalite topology.[1] We will show how periodic density functional theory (DFT) calculations offer highly accurate predictions for the thermodynamic stability of the ZIF structures as a function of linker substitution, also taking into account the effects of crystal packing of pure ligands. The accuracy of periodic DFT calculations will be backed up by the excellent correlation with the experimental solution calorimetry measurements. Moreover, it will be demonstrated how simple descriptors, such as Hammett σ-constants and electrostatic surface potentials (ESPs) offer convenient tools for rapid pre-screening of linker substituents, before performing a more in-depth computational analysis. We will continue with a demonstration of our recently-developed method for ab initio CSP, which uses the Wyckoff Alignment of Molecules (WAM) procedure to predict the structures of new MOFs, with improved computational efficiency enabled through careful consideration of molecular and crystallographic symmetry.[2] We will demonstrate the use of our CSP approach for predicting polymorphism, thermodynamic stability, porosity and optical properties of new MOFs in a series of computational studies backed up by experiment. It will also be shown how MOF CSP can be used as a tool to elucidate the crystal structures of poorly-crystalline MOFs, which are difficult to determine with X-ray diffraction methods. [1] Novendra, N., Marrett, J. M., Katsenis, A. D., Titi, H. M., Arhangelskis, M., Friščić, T. & Navrotsky, A. J. (2020). J. Am. Chem. Soc. 142, 21720. [2] Darby, J. P., Arhangelskis, M., Katsenis, A. D., Marrett, J. M., Friščić, T. & Morris, A. J. (2020). Chem. Mater. 32, 5835. External Resource: https://www.xray.cz/iucrv/vidp.asp?id=417
12:25pm - 12:45pm
Computational search for new high-TC superconductors based on lanthanoid and actinoid hydrides at moderate pressures 1Skolkovo Institute of Science and Technology, Moscow, Russian Federation; 2Dukhov Research Institute of Automatics (VNIIA); 3Moscow Institute of Physics and Technology; 4Northwestern Polytechnical University Hydrogen-rich hydrides attract great attention due to recent theoretical [1] and then experimental discovery of record high-temperature superconductivity in H3S (TC = 203 K at 155 GPa [2]). Here we perform a systematic evolutionary search for new phases in the Fe-H [3], Th-H [4], U-H [5] and other numerous systems under pressure [6] in order to predict new materials which are unique high-temperature superconductors. We predict new hydride phases at various pressures using the variable-composition search as implemented in evolutionary algorithm USPEX [7-9]. Among the Fe-H system two potentially high-TC FeH5 and FeH6 phases in the pressure range from 150 to 300 GPa were predicted and were found to be superconducting within Bardeen-Cooper-Schrieffer theory, with TC values of up to 46 K. Several new thorium hydrides were predicted to be stable under pressure using evolutionary algorithm USPEX, including ThH3, Th3H10, ThH4, ThH6, ThH7 and ThH10. ThH10 was found to be the highest-temperature superconductor with TC in the range 221-305 K at 100 GPa. Actinide hydrides show, i.e. AcH16 was predicted to be stable at 110 GPa with TC of 241 K. To continue this theoretical study, we performed an experimental synthesis of Th-H phases at high-pressures including ThH10. Obteined results can be found in Ref. [10]. Acknowledgments: This work was supported by RFBR foundation № 19-03-00100 and facie foundation, grant UMNIK №13408GU/2018. [1] D. Duan et al., Sci. Rep. 2018, 4, 6968. [2] A.P. Drozdov et al. Nature. 2015, 525, 73–76. [3] A.G. Kvashnin at al. J. Phys. Chem. C 2018, 122 4731-4736. [4] A.G. Kvashnin et al. ACS Applied Materials & Interfaces 2018, 10, 43809–43816. [5] I.A. Kruglov et al. Sci. Adv. 2018, 4, eaat9776. [6] D.V. Semenok et al. J. Phys. Chem. Lett. 2018, 8, 1920-1926. [7] A.O. Lyakhov et al. Comp. Phys. Comm. 2013, 184, 1172-1182. [8] A.R. Oganov et al. J. Chem. Phys. 2006, 124, 244704. [9] A.R. Oganov et al. Acc. Chem. Res. 2011, 44 227-237. [10] D.V. Semenok et al. 2019, Mat. Today. External Resource: https://www.xray.cz/iucrv/vidp.asp?id=418
|