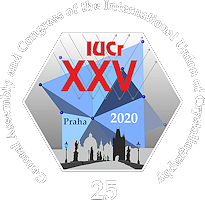
XXV General Assembly and Congress of the
International Union of Crystallography - IUCr 2021
August 14 - 22, 2021 | Prague, Czech Republic
Conference Agenda
Overview and details of the sessions of this conference. Please select a date or location to show only sessions at that day or location. Please select a single session for detailed view (with abstracts and downloads if available).
Please note that all times are shown in the time zone of the conference. The current conference time is: 26th Apr 2024, 04:36:15am CEST
|
Session Overview |
Session | ||
MS-24: Data-driven discovery in crystallography
Invited: Wenhao Sun (USA), Aria Mansouri Tehrani (Switzerland) | ||
Session Abstract | ||
The mining of large datasets and databases is now commonplace pursuit in science, and data-driven discovery has become an essential component of various fields of research (e.g., recommendation engines in materials sciences, data-driven optimization in engineering) and a significant contributing factor to their prolific output. We propose to organize a session that will focus on the promotion and integration of data-driven discovery in crystallography, with primary focus on minerals, inorganic materials, and extended inorganic solids. This session will showcase recent works that have employed large data resources, computational-driven approach, machine-learning guidance, and advanced analytical methods to realize large-scale patterns in the solid state leading to discovery. For all abstracts of the session as prepared for Acta Crystallographica see PDF in Introduction, or individual abstracts below. | ||
Introduction | ||
Presentations | ||
10:20am - 10:25am
Introduction to session 10:25am - 10:55am
Unsupervised Knowledge Discovery in ‘Big’ Materials Data University of Michigan, Ann Arbor, United States of America A major objective in recent computational materials research has been the search and discovery of novel materials with superior properties. However, prior to the availability of immense computational power, materials design was guided by conceptual frameworks for synthesis-structure-property relationships, such as Pauling’s Rules, the Hume-Rothery Rules, Pettifor Tables, Structure Maps, Ashby Tables, etc. Not only can these heuristic frameworks point us towards new and valuable materials, they also provide a satisfying conceptual foundation upon which to base our scientific intuition. In this talk, I will discuss how we can leverage unsupervised machine-learning algorithms to extract new heuristic relationships from modern large-scale materials databases. In order to extract meaningful synthesis-structure-property relationships, we will first need physically-relevant materials features. Many relevant materials features are not immediately available in current materials property databases. Determination of which features to construct will likely rely on domain knowledge and physical intuition, at least in the near-term future. We will demonstrate how these computational materials discovery and informatics tools can be used to survey, visualize, and explain stability relationships across the inorganic ternary metal nitrides.* *W. Sun et al., "A map of the inorganic ternary metal nitrides", Nature Materials (2019) 10:55am - 11:25am
Predicting ground state and metastable crystal structures using elemental and phonon mode ETH Zurich, Zurich, Switzerland We present a method to predict the crystal structure of any given composition using machine learning methods. Then, using the example of bismuth ferrite, we illustrate how crystal structure, decomposed into distortion modes, can be implemented as a feature to explore the energy surface leading to the identification of metastable polymorphs. Crystal structure plays a crucial role in determining the electronic structure and property of any composition. Therefore, it has always been of great interest to predict the crystal structure of any composition without requiring synthesis and characterization. To achieve this goal, we combine machine learning and density functional theory (DFT) calculations. Initially, a classification model predicts the point groups of the given stoichiometries. Based on the predicted point group, a series of high-throughput DFT calculations determine the ground state of non-centrosymmetric crystal structures. In addition to the ground state structure, identifying metastable polymorphs that might get stabilized by controlling the synthetic conditions is of great importance as they can exhibit different functionalities. Therefore, we studied BiFeO3 as a multifunctional compound with a rich low-energy phase space. A training set is constructed by mapping the phase space based on possible distortion modes starting from the cubic perovskite structure. A machine learning model is built using the generated training set predicting the energy surface of BiFeO3 to explore new metastable phases. Predicting ground state and metastable crystal structures using elemental and phonon mode descriptors Aria Mansouri Tehrani, Bastien Grosso, Ramon Frey, Nicola A. Spaldin Materials Theory, ETH Zurich, Wolfgang-Pauli-Strasse 27, 8093 Zürich, Switzerland aria.mansouri.t@mat.ethz.ch 11:25am - 11:45am
Beyond the constraints of chemistry: Crystal structure discovery in particle simulations 1University of Michigan, Ann Arbor, MI, USA; 2Cornell University, Ithaca, NY, USA; 3University of California, San Francisco, CA, USA; 4Argonne National Laboratory, Argonne, IL, USA; 5Friedrich-Alexander University Erlangen-Nürnberg, Erlangen, Germany Do we know all conceivable crystal structures? This question appears naive at first, because crystallography is a mature field. But the list of reported inorganic crystal structures is not necessarily representative of all kinds of order that are possible on other scales. Atomic crystal structures are affected by the discreteness of the periodic table and the resulting constraints on chemical bonding. Molecular crystals, metal organic frameworks, nanoparticle superlattices, and other soft-matter assemblies are free from these chemical constraints and can exhibit entirely new types of crystallographic order distinct from those found with atoms. A universal list of all plausible crystal structures in systems of particles ranging from the angstrom to the micrometer scale would benefit the search for—and design of—new materials. Here, we perform a data-driven simulation strategy to systematically crystallize one-component systems of particles interacting with isotropic multiwell pair potentials resembling Friedel oscillations and encoding and generalizing quantum mechanical interactions [1]. We investigate two tunable families of pairwise interaction potentials. Our simulations self-assemble a multitude of crystal structures ranging from basic lattices to complex networks. The goal is to discover crystal structures on the computer de novo, a strategy which has so far not been attempted on such a diverse set of systems. We perform a semi-automatic crystal structure analysis of simulation data. Our analysis reveals sixteen structures that have natural analogues spanning all coordination numbers found in inorganic chemistry. Fifteen more are hitherto unknown and occupy the space between covalent and metallic coordination environments. We describe the numerical search, the analysis technique, phase diagrams, and details of the known and previously unknown crystal structures. The discovered crystal structures constitute novel targets for self-assembly and expand our understanding of what a crystal structure can look like. [1] Dshemuchadse, J., Damasceno, P.F., Phillips, C.L., Engel, M., Glotzer, S.C. (2021). Proc. Natl. Acad. Sci. U.S.A. 118, e2024034118. 11:45am - 12:05pm
Data-driven approaches on pair distribution function data: matrix factorization and clustering Carnegie Mellon University, Pittsburgh, United States of America Advances in synchrotron X-ray scattering experiments have greatly increased the acquisition rates of pair distribution function (PDF) data. The analysis and interpretation of the data, however, are lagging behind the experimental advances because PDF analysis is met by the challenge of finding the correct structure model to fit against the data, which is a time-consuming process. We aim to apply data-driven methods to accelerate the analysis process of PDF data and the characterization of local material structures. Principal component analysis (PCA) and non-negative matrix factorization (NMF) are used to separate different features and/or constituents from the sample PDF data. We first applied these two methods on in-situ PDF measurement during tin oxide synthesis and then on the simulated PDFs of defected anatase titanium dioxide (TiO2). It is found that for the in-situ PDF of tin oxide synthesis, NMF is able to separate constituents during different stages of the synthesis process and their relative concentrations are consistent with the experiments. For the PDF dataset of defected anatase (TiO2), we found that NMF can separate the PDF signal of the defects from that of the perfect phase. This technique provides a tool to identify and quantify the defects from PDF data of materials. 12:05pm - 12:25pm
First-principle diffraction simulations as a tool to solve the nanodiffraction problem 1Ozyegin University, Istanbul, TURKEY; 2Columbia University, New York, USA Computer simulations are being increasingly used to understand the diffraction phenomenon from nanomaterials. Typically, such simulations are performed with the goal of establishing a mathematical relationship between the diffracting material and its diffraction profile under certain assumptions. For simulation of powder diffraction, the famous Debye equation [1] is generally used which also relies on particular assumptions about the diffracting material such as all Bragg reflections being represented by enough number of particles in the ensemble [2]. In this talk we will describe an alternative methodology that relies only on the far-field diffraction formulation [3] and starts off from the scattering phenomenon of x-rays from individual atomic positions. This methodology will be shown to be powerful and more general than the Debye equation -by relaxing some of the implicit requirements imposed by the Debye formula- enabling direct connection between each diffracted spot on a 2D detector and the diffracting crystallites [4, 5]. Once the methodology is explained, example studies on nanodiffraction experiments will be introduced and new information obtained by the computational tool will be demonstrated [6]. Although the proposed computational methodology is quite time-consuming since large number of calculations need to be performed for simulating diffraction from relatively larger nanocrystals, parallellization algorithms combined with exponentially increasing computational power becoming much more available to most researchers will potentially popularize its use in nanocharacterization studies in the near future. 12:25pm - 12:45pm
Study of noncovalent interactions using crystal structure data in the Cambridge Structural Database 1Innovation center of the Faculty of Chemistry, Belgrade, Serbia; 2Institute of Chemistry, Technology and Metallurgy, University of Belgrade, Belgrade, Serbia; 3Faculty of Chemistry, Belgrade University, Belgrade, Serbia In the recent review it was point out that the crystal structures in the Cambridge Structural Database (CSD), collected together, have contribute to various fields of chemical research such as geometries of molecules, noncovalent interactions of molecules, and large assemblies of molecules. The CSD also contributed to the study and the design of biologically active molecules and the study of gas storage and delivery [1]. In our group we use analysis of the crystal structures in the CSD to recognize and characterize new types of noncovalent interactions and to study already known noncovalent interactions. Based on the data from the CSD we can determine existence of the interactions, frequency of the interactions, and preferred geometries of the interactions in the crystal structures. In addition, we perform quantum chemical calculations to evaluate the energies of the interactions. Based on the calculated potential energy surfaces for the interactions, we can determine the most stable geometries, as well as stability of various geometries. We also can determine the interaction energies for the preferred geometries in the crystal structures. In the cases where the most preferred geometries in the crystal structures are not the most stable geometries at the potential energy surface, one can find significant influence of the supramolecular structures in the crystals. Using this methodology our group recognized stacking interactions of planar metal-chelate rings; stacking interactions with organic aromatic rings, and stacking interactions between two chelate rings. The calculated energies indicate strong stacking interactions of metal-chelate rings; the stacking of metal-chelate rings is stronger than stacking between two benzene molecules [2]. The data indicate influence of the metal and ligand type in the metal chelate ring on the strength of the interactions. Our results also indicate strong stacking interactions of coordinated aromatic rings [3]. Studies of interactions of coordinated water indicate stronger hydrogen bonds and stronger OH/π interactions of coordinated in comparison to noncoordianted water molecule [4,5]. The calculations on OH/M interactions between metal ion in square-planar complexes and water molecule indicate that these interactions are among the strongest hydrogen bonds in any molecular system [6]. The studies on stacking interactions of benzene molecules in the crystal structures in the CSD show preference for interactions at large horizontal displacements, while high level quantum chemical calculations indicate significantly strong interactions at large offsets; the energy is 70% of the strongest stacking geometry [7]. [1] Taylor, R., Wood P. A. (2019) , Chem. Rev. 119, 9427 [2] Malenov, D. P., Janjić, G. V., Medaković, V. B., Hall, M. B., Zarić, S. D. (2017) Cood. Chem. Rev. 345, 318. [3] Malenov, D. P., Zarić, S. D. (2020) Cood. Chem. Rev. 419, 213338 [4] Andrić, J. M., Janjić, G. V., Ninković, D. B., Zarić, S. D. (2012) PhysChemChemPhys, 14, 10896. [5] Andrić, J. M., Misini-Ignjatović, M. Z., Murray, J. S., Politzer. P., Zarić, S. D. (2016) ChemPhysChem. 17, 2035. [6] Janjic, G. V., Milosavljević, M., Veljković, D. Ž., Zarić S. D. (2017) Phys. Chem. Chem. Phys., 19, 8657 [7] Ninković, D. B., Blagojević Filipović, J. P., Hall, M. B., Brothers, E. N., Zarić, S. D. (2020) ACS Central Science, 6, 420. Keywords: Cambridge Structural Database; noncovalent interactions; ab initio calculations; aromatic molecules; metal complexes This work was supported by the Serbian Ministry of Education, Science and Technological Development (Contract numbers: 451-03-9/2021-14/200168 and 451-03-9/2021-14/200288) |
Contact and Legal Notice · Contact Address: Privacy Statement · Conference: IUCr 2021 | August 14 - 22, 2021 | Prague, Czech Republic |
Conference Software: ConfTool Pro 2.8.101+TC+CC © 2001–2024 by Dr. H. Weinreich, Hamburg, Germany |