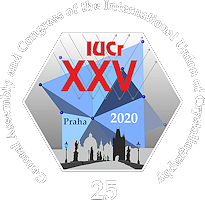
XXV General Assembly and Congress of the
International Union of Crystallography - IUCr 2021
August 14 - 22, 2021 | Prague, Czech Republic
Conference Agenda
Overview and details of the sessions of this conference. Please select a date or location to show only sessions at that day or location. Please select a single session for detailed view (with abstracts and downloads if available).
Please note that all times are shown in the time zone of the conference. The current conference time is: 1st June 2024, 02:12:42am CEST
|
Session Overview |
Session | ||
MS-17: Automation in bio-crystallography: tools, perspectives and applications
Invited: Katherine McAuley (UK), Jose Marquez (France) | ||
Session Abstract | ||
Automation in macromolecular crystallography from crystallisation, to crystal harvesting, sample mounting, data collection and data analysis as well as data management for large scale unattended and automated data collection. For all abstracts of the session as prepared for Acta Crystallographica see PDF in Introduction, or individual abstracts below. | ||
Introduction | ||
Presentations | ||
10:20am - 10:25am
Introduction to session 10:25am - 10:55am
Online Crystallography: Automated, Remote Controlled Protein-to-Structure Pipelines for Drug Design European Molecular Biology Laboratory, Grenoble, France We have developed a novel approach called CrystalDirect that enables fully automated crystal mounting and cryo-coolingclosing the automation gap between crystallization and X-ray data collection. The CrystalDirect technology also allows the automated delivery of small molecules to crystals, giving access to large scale small molecule screening through X-ray crystallography. We have combined this approach with automated data collection at the ESRF and other synchrotrons to develop a fully automated, remote-controlled pipelines for macromolecular crystallography and an automated pipeline for large scale compound and fragment screening to support structure guided drug discovery programs. In order to facilitate high throughput data analysis, we have built a series of Application Program Interfaces (APIs) linking the Crystallization Information Management System (CRIMS) and the ISPyB system for automated synchrotron data collection with automated structure refinement and analysis, using software pipelines developed by Global Phasing. These pipelines effectively provide online access to crystallization and synchrotron diffraction and data analysis facilities and remove key bottlenecks in modern crystallography. They can contribute to the rapid progression of challenging projects in structural biology, to facilitate the access to protein crystallography for scientist of other disciplines and stimulate translation of basic research into biomedical applications. On the other hand, the large amounts of data generated pose new challenges, but also provide new opportunities to develop integrated systems for data acquisition, processing and analysis. The experience from the use of these pipelines as well as the new opportunities enabled by the integration of crystallization, X-ray data collection and analysis into continuous, fully automated workflows will be discussed. 10:55am - 11:25am
Automated data collection at the Swiss Light Source macromolecular crystallography beamlines Paul Scherrer Institut, Villigen, Switzerland Crystallographic fragment screening, which involves screening small-molecule libraries against crystals of a target protein, is an essential tool in modern drug discovery. The technique relies on the high-throughput generation of cryo-cooled, soaked crystals followed by fast and efficient data collection at a synchrotron beamline. Each campaign may generate hundreds or thousands of samples, and the most efficient strategy for acquiring data is to use unattended data collection followed by automatic data processing. The macromolecular crystallography (MX) group at the Swiss Light Source operates a fast fragment and compound screening (FFCS) pipeline that uses Smart Digital User (SDU) software to collect data at the beamlines. This presentation will give an overview of SDU, describe how it has been implemented at the MX beamlines and present some recent case studies. 11:25am - 11:45am
Fully automated data collection and remote access in macromolecular crystallography beamlines at the Photon Factory, Japan 1Structural Biology Research Center, Institute of Materials Structure Science, High Energy Accelerator Research Organization, 1-1 Oho, Tsukuba, Ibaraki, Japan; 2School of High Energy Accelerator Science, The Graduate University for Advanced Studies, 1-1 Oho, Tsukuba, Ibaraki, Japan; 3Mechanical Engineering Center, Applied Research Laboratory, High Energy Accelerator Research Organization, 1-1 Oho, Tsukuba, Ibaraki, Japan Structural Biology Research Center in the Photon Factory (PF), Japan, has five macromolecular crystallography (MX) beamlines at two synchrotron radiation rings, PF and PF-AR. The end stations of all the beamlines are equipped with sample exchange robots [1], high-precision diffractometers, and pixel array detectors, which are controlled by a common control software. This enables us to realize a fully automated and unattended data collection and a remote interactive data collection at all beamlines. For a fully automated data collection, we developed a dedicated software SIROCC (Sophisticated Interface for Routine Operation with Crystal Centering). After mounting a new sample on the goniometer by the sample exchange robot, SIROCC recognizes a sample loop, and perform two raster scans over the loop regions. From a heatmap based on the number of diffraction spots below 4 Å resolution, SIROCC recognizes a shape and location of a protein crystal and places it to the X-ray beam position. By taking two snapshots, SIROCC evaluates the diffraction quality of the crystal, and if it exceeds a user’s defined threshold, SIROCC collects a complete diffraction data set. In a fully automated data collection beamtime, a beamline staff loads samples from users, and starts the automated data collection. Then all samples are mounted and diffraction measurements by SIROCC are performed in a fully automated manner. For a remote interactive data collection, NoMachine Workstation is installed on a workstation running the control software at each beamline. Furthermore, NoMachine Cloud Server, which federates the workstations at all beamlines, is installed on a gateway server which is accessible from outside the facility. At the beginning of a remote access beamtime, a beamline operating staff prepares the beamline, and give a permission for a user to access to the beamline through the gateway server. Then, the user connects through a NoMachine remote desktop software, allowing users to perform measurements remotely from outside the facility. All experimental information from the fully automated or remote data collection are recorded in the database system, PReMo [2]. PReMo also functions as a data processing and analysis pipeline, and users can obtain the experimental information and the result of data processing and analysis on the Web. Recently, a dedicated server for data download is opened, and users can download a diffraction data or data processing result to perform a further analysis with their own workstation immediately after the data collection. In remote access or fully automated data collection, the user packs frozen samples in Uni-pucks and ships them to the PF using a dry sipper. The beamline staff receives the dry sipper and transfers to the beamline which the sample is assigned. The Uni-pucks are then taken out from the dry sipper and placed in the liquid nitrogen Dewar of the sample exchange robot. To prevent miss-match of the sample mount on the diffractometer in a fully automated measurement or/and remote experiment, the transportation of the dry-shipper and placing the Uni-puck must be performed without errors. Therefore, it is very important to establish a sample tracking system for the reliable operation of fully automated measurements and remote experiments. Recently, we developed a sample tracking system for those experiments. In this system, the user must attach QR code labels to items such as a dry shipper, hard disk drive and so on to be sent, and place a pin with a two-dimensional barcode into No. 16 of the Uni-puck. When the beamline staff takes any action on the shipped items, the QR code is always read, and the status of the item is updated. The status is stored in a database system and shared among beamline staff members, which helps to prevent miscommunication. The two-dimensional barcode on the pin on No. 16 is read by the sample exchange robot after the Uni-pucks are placed in the Dewar, and the robot recognizes automatically which Uni-puck is set in which position in the Dewar. In 2020, due to the pandemic situation of COVID-19, approximately 75% of the beamtime in MX beamlines were used for remote experiments or/and fully automated measurements with users unattended. [1] Hiraki, M., Yamada, Y., Chavas, L.M., Matsugaki, N., Igarashi, N. & Wakatsuki, S. (2013). J. Phys.: Conf. Ser. 425, 012014.[2] Yamada, Y., Matsugaki, N., Chavas, L.M., Hiraki, M., Igarashi, N. & Wakatsuki, S. (2013). J. Phys.: Conf. Ser. 425, 012017. Keywords: Synchrotron, automation, remote access This research was supported by Platform Project for Supporting Drug Discovery and Life Science Research (Basis for Supporting Innovative Drug Discovery and Life Science Research (BINDS)) from AMED. 11:45am - 12:05pm
Millisecond Time-Resolved Crystallography using Film Mixing and Plunge Cooling 1Cornell University, Ithaca NY, United States of America; 2University of Waterloo, Waterloo ON, Canada Watching biomolecules in motion on biologically relevant time scales has been a long-standing goal of structural biology. Current methodologies allowing for time-resolved crystallographic data collection are mostly through serial methods using microcrystals - which are technically challenging experiments with elaborate synchrotron beamline setups, consumption of large amounts of sample, and requiring contributions from many researchers. Here, we propose an alternative methodological setup in order to collect time-resolved data in the millisecond time regime (>5ms), suitable for measuring relatively large structural changes that may be rate-limiting in particular cases. Our approach has been to leverage rapid freeze-quenching by robotically plunging our crystals of choice through a substrate film prior to hyperquenching in liquid nitrogen. This method affords many quality of life improvements over current time-resolved methods, such as the potential for a single crystal use per time-point, divorcing the reaction initiation from data collection, and the ability to use the standard mail-in remote data collection available at all synchrotron sources. In order to show proof-of-concept, we used a well characterized metabolic enzyme phosphoenolpyruvate carboxykinase which converts oxaloacetic acid to phosphoenolpyruvate. Our initial experiments uncovered a previously hypothesized state believed to occur directly after phosphoryl transfer and prior to product release. We hope that this method, with its simplicity and ease of access, can allow many structural biology labs to begin time-resolved exploration of suitable systems to uncover further molecular details of enzymes of interest. 12:05pm - 12:25pm
Automated ML-based sample centering for macromolecular X-ray crystallography with MXAimbot MAX IV Laboratory, Lund, Sweden MXAimbot is a neural network based tool, designed to automate the task of centering samples for macro-molecular X-ray crystallography experiments before exposing the sample to the beam. MXAimbot uses a convolutional neural network (CNN) trained on a few thousands images from an industrial vision camera pointed at the sample to predict suitable crystal centering for subsequent data collection. The motivation for this project is that the machine vision automated sample positioning allows X-ray laboratories and synchrotron beamlines to offer a more efficient alternative for the manual centering, which is time consuming and difficult to automate with conventional image analysis, and for the X-ray mesh scan centering, which can introduce radiation damage to the crystal. MXAimbot can be used to improve results of standard LUCID loop centering for fully automated data collection in fragment-screening campaigns. No need for sample rotation should be an additional advantage. A few original approaches and CNN architectures were tested by authors in [1,2]. They were using X-ray data from mesh scans and not relying on manual annotations. Finally for a current production a more simple method inspired by a DeepCentering approach [3] from SPring-8, has been adopted. The original training dataset was manually annotated with bounding-boxes around each crystal and the new CNN architecture is using the annotated data. MXAimbot can be used by other systems via a REST API. The next step for the project was including MXAimbot into MXCuBE3 - the common data acquisition framework at several European synchrotron facilities. This allows collection of anonymised datasets from the sample vision camera in the BioMAX beamline at the MAX IV synchrotron which can be further used for training and optimisation of CNNs and later be seamlessly included as an additional option in the MXCuBE3 data collection pipeline. To the authors knowledge CNNs have been implemented for crystal centering at least at two synchrotron facilities including MAX IV. So far the CNN approach has shown outstanding results in automatically positioning crystals. Work is currently underway to test and statistically compare the model predictions to the manual centerings by real users with the goal of integrating MXAimbot into the FragMAX [4] - fragment screening facility at the MAX IV sychrotron. [1] SCHURMANN, Jonathan; LINDHÉ, Isak. Crystal Centering Using Deep Learning. LU-CS-EX 2019-25, 2019. [2] SCHURMANN, Jonathan; LINDHÉ, Isak et al. Crystal centering using deep learning in X-ray crystallography. Asilomar Conference on Signals, Systems, and Computers, 2019, 978-983. doi: 10.1109/IEEECONF44664.2019.9048793 [3] ITO, Sho; UENO, Go; YAMAMOTO, Masaki. DeepCentering: fully automated crystal centering using deep learning for macromolecular crystallography. Journal of synchrotron radiation, 2019, 26.4: 1361-1366. doi: 10.1107/S160057751900434X [4] LIMA, Gustavo MA, et al. FragMAX: the fragment-screening platform at the MAX IV Laboratory. Acta Crystallographica Section D: Structural Biology, 2020, 76.8: 771-777. doi: 10.1107/S205979832000889X 12:25pm - 12:45pm
xia2.multiplex: a multi-crystal data analysis pipeline Diamond Light Source Ltd, Oxfordshire, United Kingdom In macromolecular crystallography, radiation damage limits the amount of data that can be collected from a single crystal. It is often necessary to merge multiple data sets from one or more crystals, for example multiple small-wedge data collections on micro-crystals, in situ room temperature data collections, lipidic mesophase data collections or time-resolved crystallography. Whilst indexing and integration of individual data sets may be relatively straightforward with existing software, additional challenges are commonly encountered when merging multiple data sets. For novel structures, identification of a consensus symmetry can be problematic, particularly in the presence of a potential indexing ambiguity. The presence of non-isomorphous or poor-quality data sets may degrade the overall quality of the merged data set. To facilitate and help optimise the scaling and merging of multiple data sets, we developed a new program, xia2.multiplex, which takes as input the results of data sets individually integrated with DIALS [1] and performs symmetry analysis [2], scaling [3] and merging of multi-crystal data sets, as well as analysis of various pathologies that typically affect multi-crystal data sets, including non-isomorphism, radiation damage [4] and preferred crystal orientation. xia2.multiplex has been deployed as part of the autoprocessing pipeline at Diamond Light Source, including integration with downstream phasing pipelines such as DIMPLE [5] and Big EP [6]. Using data sets collected as part of in situ room-temperature fragment screening experiments on the SARS-CoV-2 main protease, we demonstrate the use of xia2.multiplex within a wider autoprocessing framework to give rapid feedback during a multi-crystal experiment, and how the program can be used to further improve the quality of final merged data set. [1] Winter, G., Waterman, D. G., Parkhurst, J. M., Brewster, A. S., Gildea, R. J., Gerstel, M., Fuentes-Montero, L., Vollmar, M., Michels-Clark, T., Young, I. D., Sauter, N. K. & Evans, G. (2018). Acta Crystallographica Section D. [2] Gildea, R. J. & Winter, G. (2018). Acta Crystallographica Section D, 74(5), 405–410. [3] Beilsten-Edmands, J., Winter, G., Gildea, R., Parkhurst, J., Waterman, D. & Evans, G. (2020). Acta Crystallographica Section D, 76(4), 385–399. [4] Winter, G., Gildea, R. J., Paterson, N. G., Beale, J., Gerstel, M., Axford, D., Vollmar, M., McAuley, K. E., Owen, R. L., Flaig, R., Ashton, A. W. & Hall, D. R. (2019). Acta Crystallographica Section D, 75(3), 242–261. [5] http://ccp4.github.io/dimple/ [6] Sikharulidze, I., Winter, G. & Hall, D. R. (2016). Acta Crystallographica Section A, 72(a1), s193. |
Contact and Legal Notice · Contact Address: Privacy Statement · Conference: IUCr 2021 | August 14 - 22, 2021 | Prague, Czech Republic |
Conference Software: ConfTool Pro 2.8.102+TC+CC © 2001–2024 by Dr. H. Weinreich, Hamburg, Germany |