Research Data Management: Challenges in a Changing World
12. bis 14. März 2025 in Heidelberg und online
Veranstaltungsprogramm
Eine Übersicht aller Sessions/Sitzungen dieser Veranstaltung.
Bitte wählen Sie einen Ort oder ein Datum aus, um nur die betreffenden Sitzungen anzuzeigen. Wählen Sie eine Sitzung aus, um zur Detailanzeige zu gelangen.
|
Sitzungsübersicht |
Sitzung | ||
Präsentationen B6: Information Networks
Sitzungsthemen: Datenformate und -standards, Qualitätssicherung, Datenarchivierung, Reproduzierbarkeit und Nachnutzung, Geistes- und Sozialwissenschaften, NFDI-Bezug, Naturwissenschaften, Nicht zutreffend/Fachbereichsübergreifend
| ||
Präsentationen | ||
Knowledge Graph-based Research Data Integration for NFDI4Culture and Beyond 1FIZ Karlsruhe, Germany; 2Academy of Sciences and Literature Mainz, Germany Each NFDI consortium establishes research data infrastructures tailored to its specific domain. To facilitate interoperability across different domains and consortia, the NFDIcore ontology has been developed [1]. It serves as a mid-level ontology to represent metadata about NFDI resources, e.g. agents, projects, data portals, etc. NFDIcore establishes mappings to an array of standards across domains, including the Basic Formal Ontology, schema.org, DCTERMS, and DCAT. For domain-specific research questions, NFDIcore is extended following a modular approach, as e.g., with the NFDI-MatWerk ontology (MWO), the NFDI4DataScience ontology (NFDI4DSO), the NFDI4Memory ontology (MO), and the NFDI4Culture ontology (CTO). CTO represents resources within the NFDI4Culture domains Architecture, Musicology, Art History, Media Science, and the Performing Arts. The ontology addresses domain-specific research questions, connects diverse cultural entities, and facilitates the efficient organization, retrieval, and analysis of cultural data. The interconnection of NFDI consortia by means of Linked Open Data (LOD) opens up new research horizons. Hence, a workflow, which includes data discovery, harvesting, preprocessing, mapping, and integration into a KG is required, which is described on the use case of the NFDI4Culture KG. The NFDI4Culture KG acts as a single point of access to various decentralized research data resources and aggregates diverse and isolated data from the research domain, enabling discoverability, interoperability and reusability of CH data. The KG consists of the Research Information Graph (RIG), describing metadata such as publishers, standards, and licenses, and the Research Data Graph (RDG), interconnecting the content metadata provided by data portals. Taking into account the challenges and objectives of NFDI4Culture to aggregate a diverse landscape of CH research data, we have designed a Python package of reusable LOD components, harvesters using these components, a SPARQL endpoint explorer (shmarql), and an ETL (Extract, Transform, Load) environment[2]. The latter consists of six modular workflow components, adaptable for independent use or within a comprehensive, automated ingest routine: 1: Run harvest routines. This uses RDF-based action files with schema.org step definitions to scrape data with external tools, link the feed to its RIG metadata, and generate persistent resource identifiers. To ensure harmonization, Python-based transformations convert resources in common cultural-heritage data formats into nfdicore/cto triples when needed. 2: Clean harvested data. To ensure harmonization between the harvested data feed and its associated action file, triples representing the harvesting state are added or deleted. 3: Commit harvest state. Changes made by a harvesting run are pushed to the pipeline’s own repository to ensure up-to-date action files. 4: Prepare and index data. If there are changes in a data feed, data directories are automatically updated or created and search indexes are produced. 5: Build a new endpoint. To prevent downtimes, a new SPARQL endpoint container is built while the previous version remains available. Once the new endpoint becomes operational, the old container is stopped and removed. 6: Publish statistics. Statistics about the integrated data feeds are published in a dashboard. It supports data analysis and visualizations based on provided SPARQL queries. [1] https://ise-fizkarlsruhe.github.io/nfdicore/ [2] https://gitlab.rlp.net/adwmainz/nfdi4culture/knowledge-graph/culture-kg-kitchen Data-Driven Community Standards for Interdisciplinary Heterogeneous Information Networks 1Christian-Albrechts-Universität zu Kiel; 2Leibniz-Zentrum für Archäologie (LEIZA) Interdisciplinary research often requires merging diverse datasets into an integrated representation to reveal hidden patterns and relationships that single datasets might not show. Heterogeneous Information Networks (HINs) (Sun et al., 2011) offer a flexible framework for integrating various datasets. They preserve both the data’s complex relationships and contextual information in an intuitively understandable graph structure containing typed nodes and edges. In our work, we aim to integrate archaeological data from the Big Exchange project (Kerig et al., 2023) with ancient DNA data from the Poseidon project (Schmid et al., 2024), utilizing HINs to enable deeper analysis through shared spatial and temporal concepts. However, we require standards to ensure semantically correct integration of HINs. The following two types of standards are crucial to ensure that integrated datasets maintain their semantic integrity and are accurately linked. • Schema-level standards: Network schemata, defining the types of objects and their relationships, must be aligned, i.e., the same real-world concepts are represented consistently across different network schemata. Standardized ontologies may provide a unified conceptual framework to enable semantic integration of HINs at schema-level. • Data-level standards: Data consistency is essential to ensure that multiple instances of the same real-world entity are consistently mapped to the same object. This process, known as entity resolution, is crucial to successfully merge datasets. Authority files and controlled vocabularies may provide standardized representations for entity resolution. We use the CIDOC Conceptual Reference Model, "a formal ontology intended to facilitate the integration, mediation and interchange of heterogeneous cultural heritage information and similar information from other domains" (Bekiari et al., 2021), to assure schema-level consistency. We then aim to ensure data-level consistency by proposing suitable controlled vocabularies and authority files covering the represented domains, facilitating the mapping of diverse representations of the same real-world entities to unified, consistent nodes. This process results in a semantically consistent, integrated HIN, enhancing the potential to uncover novel patterns and insights between the integrated data that remain hidden when the datasets are viewed in isolation. This research aligns with the conference by promoting research data standards that enhance interoperability of heterogeneous datasets in an interdisciplinary context. Our adherence to schema- and data-level standards aligns with the FAIR principles, ensuring that the integrated HINs are not only semantically consistent but also interoperable and reusable for future research. By sharing our approach, we aim to contribute to broader efforts that promote data interoperability and semantic consistency of heterogeneous datasets, both within cultural heritage and across other interdisciplinary research fields. References Bekiari, C., Bruseker, G., Doerr, M., Ore, C.-E., Stead, S., and Velios, A. (2021). ‘Definition of the CIDOC Conceptual Reference Model v7.1.1 (Version v7.1.1).’. Kerig, T., Hilpert, J., Strohm, S., et al. (2023). ‘Interlinking research: the Big Exchange project’. Schmid, C., Ghalichi, A., Lamnidis, T. C., Mudiyanselage, D. B. A., Haak, W., and Schiffels, S. (2024). 'Poseidon – A Framework for Archaeogenetic Human Genotype Data Management’. Sun, Y., Han, J., Yan, X., Yu, P., and Wu, T. (2011). ‘PathSim: Meta Path-Based Top-K Similarity Search in Heterogeneous Information Networks’. |
Impressum · Kontaktadresse: Datenschutzerklärung · Veranstaltung: E-Science-Tage 2025 |
Conference Software: ConfTool Pro 2.8.106+CC © 2001–2025 by Dr. H. Weinreich, Hamburg, Germany |
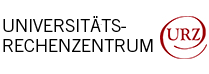